A/B testing is a robust process that allows you to compare two versions of a landing page or element, enabling you to identify which version performs better and drives more conversions.
This comprehensive article will delve deep into A/B testing and explore how to leverage your outcomes to optimize your landing pages. Whether you’re a marketer with a background in statistics or someone looking to take their A/B testing strategies to the next level, this article will arm you with the essential skills and knowledge to conduct practical experiments that result in more data-driven decisions.
A/B testing is a robust process that allows you to compare two versions of a landing page or element, enabling you to identify which version performs better and drives more conversions. By conducting controlled A/B experiments and analyzing user behavior, you can gather valuable insights into the impact of your marketing operations and make informed decisions to optimize your landing pages. To get the most out of this guide, we recommend having a basic understanding of statistics. However, even if you’re new to the field, we’ll provide explanations and examples to help you grasp the key concepts.
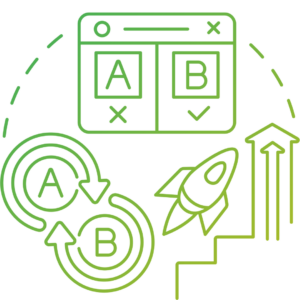
Step 1: Understanding A/B Testing
A/B testing, also known as Split Testing, is a technique used to compare two versions of a landing page or element to determine which one performs better and drives more conversions. Marketers can make better, data-driven decisions and optimize their web pages for better results by conducting controlled experiments. A/B testing allows you to test variables and variations, measure their impact, and identify the most effective strategies and builds to achieve your goals.
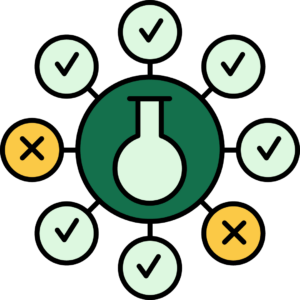
Step 2: Defining Your Hypothesis
Clear hypotheses are essential for a successful A/B test. They provide a framework for your experiment and help you define specific changes or variations you want to test.
Formulating testable hypotheses involves:
When defining hypotheses for your A/B test, you must consider the specific goals you want to achieve. For example, if your goal is to increase click-through rates (CTR) on a landing page, your hypothesis could be that changing the color of the call-to-action button will lead to a higher click-through rate. By formulating clear ideas, you can create a structured experiment and measure the impact of specific changes made.
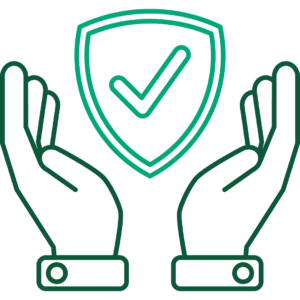
Step 3: Implementing Your A/B Test
Choosing the right testing platform is essential for implementing your A/B test. There are various tools available that offer features for designing, launching, and monitoring tests. Once you have selected a platform, you must set up your test by specifying the variations and defining the target audience. Proper tracking and data collection methods should also be implemented to ensure accurate measurement and analysis.
Implementing your A/B test involves setting up the variations and defining the target audience for your experiment. This could include creating different versions of a webpage or element using a testing platform and specifying the percentage of users that will be exposed to each version. It is vital to ensure proper tracking and data collection methods to accurately measure the performance of each variation and analyze the results.
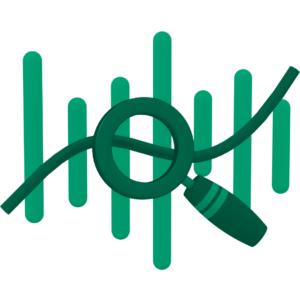
Step 4: Collecting and Analyzing Data
During the A/B test, it is crucial to track and measure key metrics to evaluate the performance of each variant. Start by identifying and tracking key metrics such as click-through rates between pages, bounce rates out of your landing page, and conversion rates. Statistical techniques, such as calculating statistical significance and determining sample size, are used to ensure the reliability of the results. These statistical models will help you identify if there are substantial differences between the variations and draw meaningful conclusions.
To ensure the reliability of your results collected, make sure your test checks all statistical bases: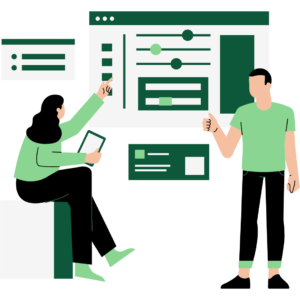
Step 5: Interpreting Results and Drawing Conclusions
Interpreting the results of an split test involves comparing the performance of the different variations and analyzing the data collected. By calculating confidence intervals, marketers can determine the level of certainty in their results and make informed decisions based on statistical significance.
The confidence interval provides a range of values around the estimated metric value that is likely to contain the true value with a certain level of confidence. The confidence level, often expressed as a percentage (e.g., 95% confidence level), indicates the probability that the true value falls within the interval.
For example, if you have an A/B test comparing two variations of a webpage and calculate a conversion rate of 10% for Variation A with a 95% confidence interval of ±2%, it means that you can be 95% confident that the true conversion rate of Variation A falls within the range of 8% to 12%.
A wider confidence interval indicates more uncertainty in the estimate, while a narrower interval suggests greater precision. When the confidence intervals of two variations do not overlap, it suggests a statistically significant difference between them. On the other hand, if the intervals overlap, it indicates that the observed difference may be due to chance, and the results are not statistically significant.
Drawing meaningful conclusions from the A/B test allows marketers to gain insights into user preferences, identify effective strategies, and optimize their landing pages to improve conversion rates.
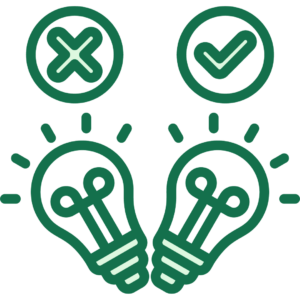
Step 6: Iterating and Optimizing
A/B testing is an iterative process. To build a healthy process, consider the below tips:
Conclusion: Onwards and Upwards
Following the steps outlined in this advanced article, you will be equipped with the more profound knowledge and vital skills to A/B test your landing pages effectively. As you iterate the A/B testing process, you will embark on a continuous journey towards building a user experience that methodologically surfs the waves of trends and effectively achieves the goals you’ll be striding for.
Do you have any questions on how to launch your next successful A/B Test? At VANE IVY, we use our extensive background in data and marketing to help you and your team to expedite your process. Give us a shout!