Well-processed data now underpins the decisions that drive successful marketing campaigns, build effective strategies, and shape customer interactions.
Table of Contents
Data Ontology: Unpacking the Concept
Ontology is a unique conceptual framework that defines and organizes data, particularly marketing data. The term ‘ontology’ originates from philosophy, which refers to the study of existence but has since been adopted in various fields, including data management. In this context, ontology assumes a practical significance and is pivotal in understanding and using data.
In its essence, ontology provides a lifeline in the often overwhelming and vast sea of data. It brings structure and order, cutting through the chaos and providing a system for understanding data, its interrelationships, and its implications. Ontology can be likened to a roadmap that provides clear, understandable paths through the complex maze of data, making it easier to navigate and extract value.
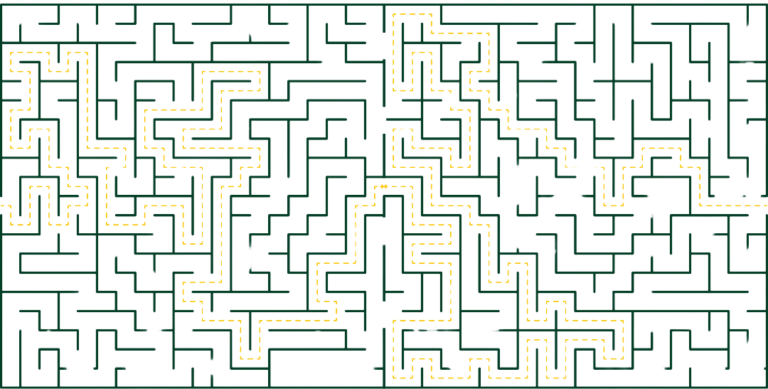
By providing structure and context to data, ontologies make it more comprehensible and usable. They offer a way to classify and organize data to reflect its inherent meaning and relationships. This improves the accuracy and relevancy of data analysis and significantly enhances the decision-making process.
To further delve deeper and expand on this concept, let’s explore the four pillars that make up your data ontology:
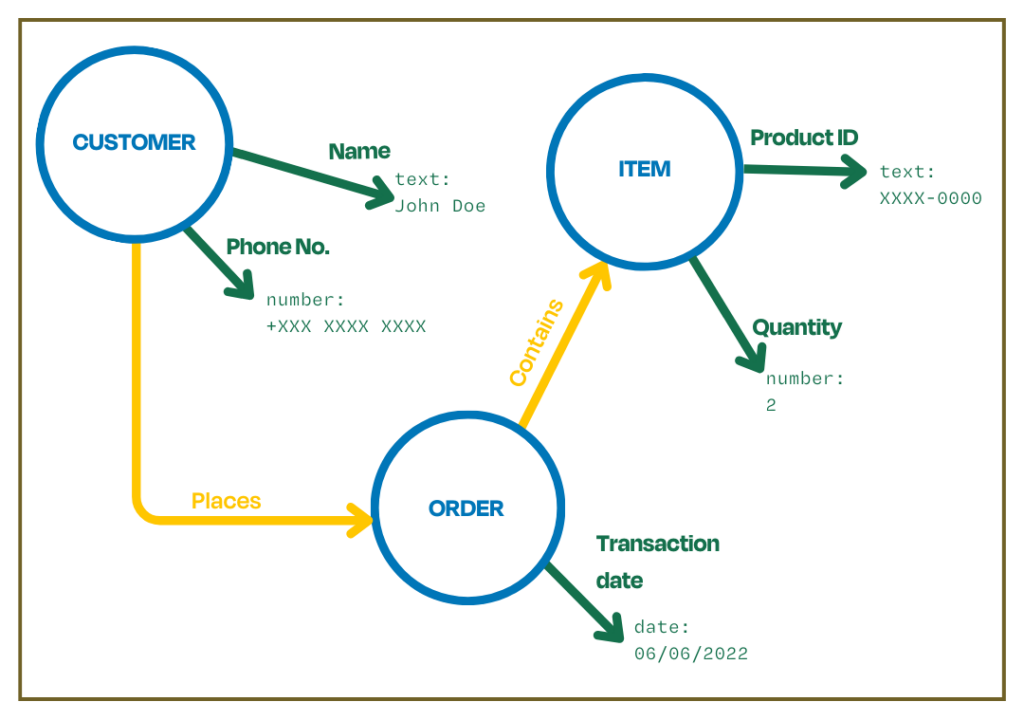
Classes / Concepts
In a marketing data ontology, concepts or classes represent the main entities or objects under consideration. This could include Customers, Products, Transactions, Shopping Carts, and Campaigns, among others. These classes form the building blocks of the ontology, representing the critical aspects of the business. Here are some examples to spark your imagination:
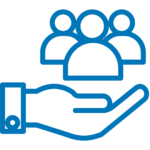
Customers
Attributes of the ‘Customers’ class might include ‘Customer_ID,’ ‘Name,’ ‘Email_Address,’ ‘Location,’ ‘Age,’ etc.
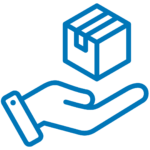
Products
This class encompasses all items or services sold by the business. Each product listed for sale on the website would be an instance in this class.
Attributes might include ‘Product_ID,’ ‘Product_Name,’ ‘Product_Description,’ ‘Price,’ ‘Stock_Level,’ etc.

Transactions
This class represents all purchases made by customers. Each instance would be a specific transaction in which a customer purchased one or more products.
Attributes might include ‘Transaction_ID,’ ‘Customer_ID,’ ‘Total_Amount,’ ‘Transaction_Date,’ etc.
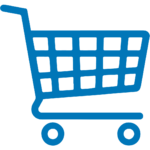
Shopping Carts
This class includes all active and abandoned shopping carts. Each instance would be a specific shopping cart containing one or more products added by a customer.
Attributes might include ‘Cart_ID,’ ‘Customer_ID,’ ‘Total_Items,’ ‘Total_Value,’ etc.
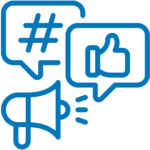
Campaigns
This class represents all marketing campaigns run by the business. Each instance would be a specific campaign to promote the business or its products.
Attributes might include ‘Campaign_ID,’ ‘Campaign_Name,’ ‘Start_Date,’ ‘End_Date,’ ‘Target_Audience,’ etc.
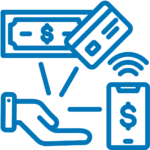
Payment Methods
This class represents how customers can pay for their purchases. Each instance would be a specific payment method available to customers.
Attributes might include ‘Payment_Method_ID,’ ‘Payment_Method_Name,’ (e.g., ‘Credit Card,’ ‘PayPal,’ ‘Cash on Delivery’), ‘Transaction_Fees,’ etc.
Other categories can be added, or some of the above can be dropped. These can be tailored to each business type seeking to launch a data ontology. By categorizing the different types of classes, you can then move to explore the relationships that govern interactions between them.
Properties / Attributes
Attributes or properties provide more detailed information about the classes in the ontology. These attributes help add depth to the classes, allowing for more detailed analysis and insights and can be classified into several categories. Here are some examples::
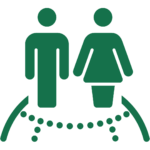
Customer Demographics
This includes information about the customer’s characteristics, such as age, location, gender, occupation, and income level. This data can help businesses understand their target audience and tailor their marketing strategies accordingly.
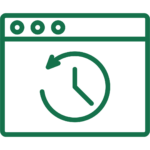
Customer Behavior
This involves data related to the customer’s interactions with the business, such as purchase history, browsing history, product preferences, and response to marketing campaigns. It can provide insights into customer preferences and behavior, helping businesses to improve their products, services, and marketing efforts.

Campaign Metrics
These variables are related to the performance of marketing campaigns. They include metrics like click-through rates, conversion rates, bounce rates, and customer acquisition costs. Analyzing these metrics can help businesses evaluate the effectiveness of their marketing strategies and make necessary adjustments.
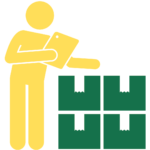
Product Data
Involves information about the business’s products or services. It can include data on sales, stock levels, returns, and customer reviews. This data can evaluate product performance, identify popular products, and manage inventory effectively.
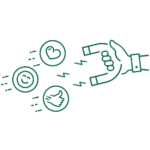
Performance metrics
Measure the overall performance of a business’s online presence. They can include website traffic, page views, session duration, social media engagement, and email open rates. By analyzing these metrics, businesses can assess their online performance and identify areas for improvement.
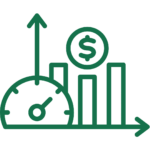
Financial Data
These are variables related to the business’s economic performance. They can include revenue, costs, profitability, return on investment, and other financial measures. This data can be used to evaluate the financial performance of marketing strategies and make informed business decisions. These are just some variables and metrics that can be included in a marketing ontology. The specific variables and metrics used can vary greatly depending on the nature of the business and its marketing objectives.
By categorizing the different types of classes and identifying the attributes collected under each class, you can explore the relationships that govern interactions between classes, and the class-bound attributes that populate your data ontology landscape. Let’s take a look at the relationships that can start forming.
Relationships
Relationships link the different classes in the ontology and define how they interact. They are crucial in understanding business dynamics and revealing patterns and trends that can inform strategic decision-making. In a marketing ontology, several types of relationships might be defined. Let’s explore some of these potential relationships:

Customers purchase products
This relationship is at the heart of every business. It represents the transactional interaction between customers and the products or services they buy. This relationship can provide insights into customer preferences, popular products, and sales trends.
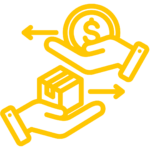
Products included in transactions
This relationship illustrates the association between individual products and specific sales transactions. It can provide valuable data on which products are most often purchased together, which can inform strategies such as product bundling or recommendations.
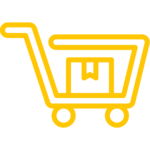
Shopping cart contains products
This relationship depicts the contents of a customer’s shopping cart before making a purchase. It can help businesses understand which products customers are considering and identify points in the purchasing process where customers may abandon their shopping cart.
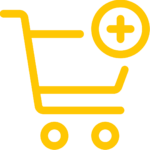
Customers add products to the shopping cart
This signifies the action of a customer placing a product in their shopping cart. Analyzing this relationship can provide insights into customer behavior and preferences and help identify popular products.
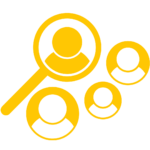
Customers belong to segments
This relationship categorizes customers into segments based on demographics, past purchase behavior, or engagement with marketing campaigns. Understanding this relationship can enable more personalized and effective marketing strategies.
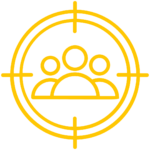
Marketing campaigns target customer segments
This relationship indicates which marketing campaigns are aimed at which customer segments. Examining this relationship can help assess the effectiveness of marketing campaigns and can guide future marketing strategies by identifying which campaigns resonate with which segments.
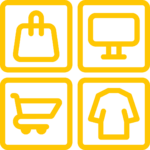
Products belong to categories
This relationship groups products into different categories, which can help businesses manage their product offerings and provide customers with a more organized shopping experience.
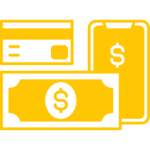
Transactions categorized by payment type
This relationship sorts transactions based on how customers pay, such as with credit card, PayPal, or cash on delivery. Understanding this relationship can help businesses identify popular payment methods and ensure they meet their customers’ needs.
These relationships, among other things, help map out the complex interactions between different entities within a business. They are crucial in understanding the business dynamics and can provide valuable insights that inform marketing strategies.
Instances
Instances are the specific examples or occurrences that belong to each class. For example, each customer would be an instance in the Customers’ class, and each product sold would be an instance in the Products’ class. Instances bring the ontology to life, populating it with real-world data.
For inspiration, here’s some other examples we can list out:
Customers
John Doe, a 35-year-old man from New York who frequently buys electronics, can be considered an instance of the ‘Customers’ class. Another instance could be Jane Smith, a 25-year-old woman from California who often purchases books.
Products
An iPhone 12 with a price of $699 and a stock level of 200 units can be seen as an instance of the ‘Products’ class. Another instance could be a hardcover copy of “Pride and Prejudice”, priced at $15 with 500 units in stock.
Transactions
A specific purchase made by John Doe on January 1, 2022, where he bought an iPhone 12 and paid $699, is an instance of the ‘Transactions’ class. Another instance could be a purchase made by Jane Smith on February 15, 2022, where she bought a “Pride and Prejudice” book and paid $15.
Shopping Carts
A shopping cart created by John Doe on March 1, 2022, containing an iPhone 12 and a total value of $699, is an instance of the ‘Shopping Carts’ class. Another instance could be a shopping cart abandoned by Jane Smith on March 15, 2022, containing a “Pride and Prejudice” book and a total value of $15.
Campaigns
A spring sale campaign running from March 20, 2022, to April 20, 2022, targeting all customers and promoting a 10% discount on all products, can be considered an instance of the ‘Campaigns’ class.
Payment Methods
Different payment methods like ‘Credit Card,’ ‘PayPal,’ and ‘Cash on Delivery’ can be considered as instances of the ‘Payment Methods’ class. For example, ‘Credit Card’ as a payment method with a transaction fee of 2% is an instance..
Adding these instances to the marketing data ontology, we populate it with real-world data, bringing the ontology to life. These instances when carefully considered and defined, help in creating a more comprehensive, structured framework for managing and analyzing marketing data. They help clarify the intricate web of interactions and relationships within the business, making the data more manageable, comprehensible, and, ultimately, more valuable.
Examining the Importance of Ontology for Marketing Executives
Understanding and implementing ontology in marketing data management can yield many significant benefits. It offers a unified, standardized approach to understanding data, which can streamline communication across different teams. This proves particularly beneficial in larger organizations where multiple teams may work with the same data but interpret it differently.
Ontology provides a standardized understanding of data, minimizing misinterpretations and fostering collaboration. It offers a common language that ensures everyone is on the same page, promoting clarity and consistency in data interpretation across the organization.
Furthermore, ontologies can significantly enhance data analysis. By providing context and interconnections between data points, they can help identify patterns and trends that might go unnoticed. This can lead to more informed and strategic decisions and, ultimately, better business outcomes.
Evaluating the Pros and Cons
While the advantages of ontology are clear, it’s also important to acknowledge its challenges. On the positive side, the primary advantage of ontology is the clarity and structure it brings to data. It can make data analysis more effective, streamline communication, and foster better decision-making. By providing a structured approach to data, ontology can help businesses unlock the full potential of their data.
However, building and maintaining an ontology requires considerable time and resources. It’s not a one-time effort but an ongoing process that demands commitment. Furthermore, it requires a deep understanding of the business and the data it generates. Effective ontology creation involves a blend of technical expertise and business acumen.
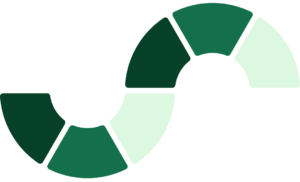
How to Build your Marketing Data Ontology
A Detailed Step-by-Step Guide to Building a Marketing Data OntologyDeveloping an ontology for marketing data may initially seem daunting, but it doesn’t have to be. It’s a systematic process that can be broken down into manageable steps:
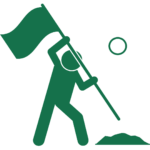
Establish Goals
Clearly define the objectives and aims of crafting a data ontology. Ensure these align with the overarching goals of the business domain for which the ontology will be built. For instance, if you are building a marketing data ontology, the domain would be marketing, and the objective could be to improve the organization and analysis of marketing data to improve campaign performance.

Assemble Team
Bring together domain experts, data analysts, data architects, and other relevant stakeholders. Multi-department collaboration ensures that the ontology comprehensively and accurately represents the domain while nurturing the required builder skills to determine what is attainable. For instance, building a marketing data ontology could involve marketing executives deciding the purpose for which channel data needs to be collected, while data scientists and developers can help guide how to collect and properly store that data.
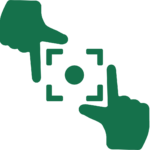
Set Scope and Limits
Define the breadth and depth of the ontology, outlining which concepts and relationships will be incorporated. Establish boundaries to prevent the ontology from becoming excessively broad or complex. This would involve deciding what aspects of marketing data to include, such as customer data, product data, transaction data, etc, pinned by data privacy laws and ethics.
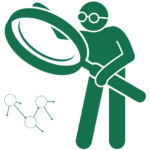
Identify Key Concepts
The first step in building your ontology is identifying the key concepts related to your data. Identify their attributes, properties, and relationships. In the context of a marketing data ontology, these might include customers, campaigns, products, or other relevant entities. This step is crucial as the concepts you identify will serve as the foundation of your ontology.
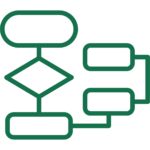
Define Relationships
Once you have identified the concepts, the next step is to define how they relate. These relationships form the backbone of your ontology. For instance, in a retail business, a critical relationship could be ‘customers purchase products’.

Create Hierarchies
This involves organizing your concepts into hierarchies. This helps in understanding the broader context and the specific details. A hierarchy could be as simple as ‘products are categorized into different types’ or more complex, depending on the nature of your data.

Implement the Ontology
After crafting your ontology, the next step is to integrate it into your data management systems. Choose an ontology language (e.g., RDF, OWL—more in the section below) to formalize the ontology. Use tools like Graph DB or Protégé to create and visualize the ontology. It’s worth noting that even more straightforward tools like Visio can also be practical.
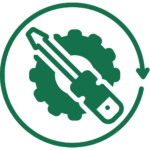
Maintain and Update
An ontology is not a static creation. As your business and data needs evolve, so should your ontology. Regular maintenance and updates are necessary to ensure your ontology remains relevant and practical.
Deep Insight on RDF and OWL for Ontology
The Resource Description Framework (RDF) and the Web Ontology Language (OWL) are integral in creating and implementing ontologies, particularly in semantic web technologies.
DF (Resource Description Framework) is a standard model for data interchange on the web. It provides a straightforward way to express data in the form of subject-predicate-object triples, allowing for the definition of explicit relationships and the creation of a linked data structure.Each RDF triple consists of a subject, a predicate, and an object. The subject is the resource being described, the predicate is the aspect or attribute of the subject being expressed, and the object is the value of that attribute. For example, in a marketing context, the subject could be a ‘Product,’ the predicate could be ‘price,’ and the object could be ‘$20’. This forms a triple that expresses a specific aspect of the product.
OWL (Web Ontology Language), conversely, is a semantic web language designed to represent rich and complex knowledge about things, groups of things, and relations between things. It is a computational logic-based language that allows users to make explicit semantic assertions about objects and their relationships OWL is particularly well-suited for creating ontologies due to its expressive power and ability to define classes, properties, and relationships. It allows for a high level of definition and specificity, including the ability to express characteristics such as cardinality, symmetry, and transitivity, among others. In a marketing data ontology, RDF could express the data relationships, while OWL could define the classes and properties of the ontology. For example, OWL could represent a ‘Customer’ class and its properties (like ‘hasAge’ and ‘hasLocation’), while RDF could express specific customer data (like ‘Customer123 hasAge 30’).
RDF and OWL provide powerful tools for creating, implementing, and managing ontologies. They allow for the detailed definition, expression, and linking of data, making them invaluable in marketing data management.
Concluding Thoughts
A well-structured marketing data ontology can be a powerful tool for leveraging data in digital marketing. It can streamline processes, improve communication, and enhance data analysis. However, like any tool, it should be used thoughtfully and adjusted as needed. As the world of data continues to expand phenomenally, the role of ontology in managing and interpreting this data will only become more crucial.
Therefore, it’s time to embrace ontology and unlock its potential to transform your marketing data management. As you embark on this journey, remember that the goal is to manage data and make it work for you, driving your marketing strategies and, ultimately, your business success. In the end, the power of ontology lies not just in taming the chaos of data but in harnessing it to create meaningful, actionable insights that can propel your business forward.
Do you have any questions on how to embark on better marketing data management? At VANE IVY, we use our extensive background in data and marketing to help you and your team to expedite your build process. Give us a shout!